How Yieldmo Cut Database Costs and Cloud Dependencies
Rethinking latency-sensitive DynamoDB apps for multicloud, multiregion deployment “The entire process of delivering an ad occurs within 200 to 300 milliseconds. Our database lookups must complete in single-digit milliseconds. With billions of transactions daily, the database has to be fast, scalable, and reliable. If it goes down, our ad-serving infrastructure ceases to function.” – Todd Coleman, technical co-founder and chief architect at Yieldmo Yieldmo’s online advertising business depends on processing hundreds of billions of daily ad requests with subsecond latency responses. The company’s services initially depended on DynamoDB, which the team valued for simplicity and stability. However, DynamoDB costs were becoming unsustainable at scale and the team needed multicloud flexibility as Yieldmo expanded to new regions. An infrastructure choice was threatening to become a business constraint. In a recent talk at Monster SCALE Summit, Todd Coleman, Yieldmo’s technical co-founder and chief architect, shared the technical challenges the company faced and why the team ultimately moved forward with ScyllaDB’s DynamoDB-compatible API. You can watch his complete talk below or keep reading for a recap. Lag = Lost Business Yieldmo is an online advertising platform that connects publishers and advertisers in real time as a page loads. Nearly every ad request triggers a database query that retrieves machine learning insights and device-identity information. These queries enable its ad servers to: Run effective auctions Help partners decide whether to bid Track which ads they’ve already shown to a device so advertisers can manage frequency caps and optimize ad delivery The entire ad pipeline completes in a mere 200 to 300 milliseconds, with most of that time consumed by partners evaluating and placing bids. More specifically: When a user visits a website, an ad request is sent to Yieldmo. Yieldmo’s platform analyzes the request. It solicits potential ads from its partners. It conducts an auction to determine the winning bid. The database lookup must happen before any calls to partners. And these lookups must complete with single-digit millisecond latencies. Coleman explained, “With billions of transactions daily, the database has to be fast, scalable and reliable. If it goes down, our ad-serving infrastructure ceases to function.” DynamoDB Growing Pains Yieldmo’s production infrastructure runs on AWS, so DynamoDB was a logical choice as the team built their app. DynamoDB proved simple and reliable, but two significant challenges emerged. First, DynamoDB was becoming increasingly expensive as the business scaled. Second, the company wanted the option to run ad servers on cloud providers beyond AWS. Coleman shared, “In some regions, for example, the US East Coast, AWS and GCP [Google Cloud Platform] data centers are close enough that latency is minimal. There, it’s no problem to hit our DynamoDB database from an ad server running in GCP. However, when we attempted to launch a GCP-based ad-serving cluster in Amsterdam while accessing DynamoDB in Dublin, the latency was far too high. We quickly realized that if we wanted true multicloud flexibility, we needed a database that could be deployed anywhere.” DynamoDB Alternatives Yieldmo’s team started exploring DynamoDB alternatives that would suit their extremely read-heavy database workloads. Their write operations fall into two categories: A continuous stream of real-time data from their partners, essential for matching Yieldmo’s data with theirs Batch updates driven by machine learning insights derived from their historical data Given this balance of high-frequency reads and structured writes, they were looking for a database that could handle large-scale, low-latency access while efficiently managing concurrent updates without degradation in performance. The team first considered staying with DynamoDB and adding a caching layer. However, they found that caching couldn’t fix the geographic latency issue and cache misses would be even slower with this option. They also explored Aerospike, which offered speed and cross-cloud support. However, they learned that Aerospike’s in-memory indexing would have required a prohibitively large and expensive cluster to handle Yieldmo’s large number of small data objects. Additionally, migrating to Aerospike would have required extensive and time-consuming code changes. Then they discovered ScyllaDB, which also provided speed and cross-cloud support, but with a DynamoDB-compatible API (Alternator) and lower costs. Coleman shared, “ScyllaDB supported cross-cloud deployments, required a manageable number of servers and offered competitive costs. Best of all, its API was DynamoDB-compatible, meaning we could migrate with minimal code changes. In fact, a single engineer implemented the necessary modifications in just a few days.” ScyllaDB evaluation, migration and results To start evaluating how ScyllaDB worked in their environment, the team migrated a subset of ad servers in a single region. This involved migrating multiple terabytes while keeping real-time updates. Process-wise, they had ScyllaDB’s Spark-based migration tool copy historical data, paused ML batch jobs and leveraged their Kafka architecture to replay recent writes into ScyllaDB. Moving a single DynamoDB table with ~28 billion objects (~3.3 TB) took about 10 hours. The next step was to migrate all data across five AWS regions. This phase took about two weeks. After evaluating the performance, Yieldmo promoted ScyllaDB to primary status and eventually stopped writing to DynamoDB in most regions. Reflecting on the migration almost a year later, Coleman summed up, “The biggest benefit is multicloud flexibility, but even without that, the migration was worthwhile. Database costs were cut roughly in half compared with DynamoDB, even with reserved-capacity pricing, and we saw modest latency improvements. ScyllaDB has proven reliable: Their team monitors our clusters, alerts us to issues and advises on scaling. Ongoing maintenance overhead is comparable to DynamoDB, but with greater independence and substantial cost savings.” How ScyllaDB compares to DynamoDBScyllaDB Cloud: Fully-Managed in Your Own Google Cloud Account
You can now run ScyllaDB’s monstrously fast and scalable NoSQL database within your own Google Cloud (GCP) accounts We’re pleased to share that ScyllaDB Cloud is now available with the Bring Your Own (Cloud) Account model on Google Cloud. This means: ScyllaDB runs inside your private Google Cloud account. Your data remains fully under your control and never leaves your Google Cloud account. Your database operations, updates, monitoring, and maintenance are all managed by ScyllaDB Cloud. Existing cloud contracts and loyalty programs can be applied to your ScyllaDB Cloud spend. This is the same deployment model that we’ve offered on AWS for nearly 4 years. The BYOA model is frequently requested by teams who want both: The fully managed ScyllaDB Cloud service with near-zero operations and maintenance. The regionality, governance, and billing benefits that come from running in your private cloud account It’s especially well-suited for highly regulated industries like healthcare and finance with Data privacy, compliance, and data sovereignty guarantees. With BYOA, all ScyllaDB servers, storage, networking, and IP addresses are created in your cloud account. Data never leaves your VPC environment; all database resources remain under your ownership and governance policies. For additional security, ScyllaDB Cloud runs Bring Your Own Key (BYOK), our transparent database-level encryption, encrypting all the data with CMK. If you are the target of a cyberattack, or you have a security breach, you can protect the data immediately by revoking the database key. Under the BYOA model, the infrastructure costs are paid directly to the cloud provider. That means your organization can apply its existing GCP commitments and take advantage of any available discounts, credits, or enterprise agreements (e.g., Committed Use, Sustained Use, Enterprise Agreements(EA)). ScyllaDB Cloud costs are reduced to license and support fees.NOTE: The Bring Your Own (Cloud) Account feature is often addressed as BYOC, spotlighting the “Cloud” aspect. We prefer the term “account” as it more accurately represents our offering, though both concepts are closely related.How ScyllaDB BYOA Works on Google Cloud Once BYOA service is enabled for your GCP project , the ScyllaDB Cloud control plane can use the Google Cloud API to create the necessary resources in your designated GCP project. After the network is configured, ScyllaDB Cloud securely connects to your cluster’s VPC to provision and manage ScyllaDB database clusters. You can configure a VPC peering connection between your application VPC and your ScyllaDB dedicated cluster VPC (as shown on the right side of the diagram). Our wizard will guide you through the configuration process for your GCP project. Using the wizard, you will configure one IAM role with policies to provision the required resources within the GCP project. ScyllaDB Cloud will operate using this role. Configuration To use the Bring Your Own Account feature, you will need to choose one project in your GCP account. This project will be used as a destination to provision your clusters. The specific policies required can be found here. Make sure your Cloud quotas are as per the recommendation. Here’s a short guide on how you can configure your GCP account to work with ScyllaDB Cloud. You will need permissions to a GCP account and a very basic understanding of Terraform. Once you complete the setup, you can use your GCP Project as any other deployment target. In the create new cluster screen, you can select this project next to ScyllaDB Cloud hosted option. In the “Create New Cluster” screen, you will be able to select this project alongside the ScyllaDB Cloud hosted option. You can select a geographical area (Region), the nature of access (private/public), and the expected instance type based on the volume of traffic, ScyllaDB Cloud will create a ScyllaDB cluster for you. From there, you can choose a geographical region, specify the type of access (public or private), and select the appropriate instance type based on your expected traffic volume. ScyllaDB Cloud will then provision and configure a cluster for you accordingly. Next steps ScyllaDB Cloud BYOA is currently live on Google Cloud Platform. If you’re ready to set up your account, you can go to http://cloud.scylladb.com to use our onboarding wizard and our step-by-step documentation. Our team is available to support you — from setup to production. Just ping your existing representative or reach out via forums, Slack, chat, etc.
Why DynamoDB Costs Catch Teams Off Guard
From inevitable overprovisioning to the “on-demand” tax: why DynamoDB is bloody hard to cost-control I recently built a DynamoDB cost calculator with the specific goal of helping potential ScyllaDB customers understand the true cost of running DynamoDB. Now, if you step back and look at my goal, it doesn’t make much sense, right? If somebody is already using DynamoDB, wouldn’t they already know how much it costs to run the technology at scale? Naively, this is what I thought too, at first. But then, I started to peel back the inner workings of DynamoDB cost calculations. At that point, I realized that there are many reasons why teams end up paying hundreds of thousands (if not millions) of dollars to run DynamoDB at scale. The main thing I found: DynamoDB is easy to adopt, but bloody hard to cost-control. My workmate Guilherme and I delivered a webinar along these lines, but if you don’t have time to watch, read on to discover the key findings. The first common misunderstanding is precisely what DynamoDB charges you for. You’ve probably already heard terms like Read Capacity Units and Write Capacity Units, and get the gist of “You pay for what you use” in terms of number of reads and writes. But let’s start with the basics. DynamoDB writes are expensive… If you look at pricing for on-demand capacity, you’ll see that a read request unit (RRU) costs $0.125 per million units, and a write request unit (WRU) costs $0.625 per million units. So, writes are 5 times more expensive than reads. I don’t know the exact technical reason, but it’s no doubt something to do with the write path being heavier (durability, consistency, indexing etc) and perhaps some headroom. 5x does seem a bit on the steep side for databases and one of the first traps from a cost perspective. You can easily find yourself spending an order of magnitude more if your workload is write-heavy, especially in on-demand mode. Speaking of which…there’s the other mode: provisioned capacity. As the name suggests, this means you can specify how much you’re going to use (even if you don’t use it), and hopefully pay a bit less. Let’s check the ratio though. A Read Capacity Unit (RCU) costs $0.00013 per RCU and a Write Capacity Unit (WCU) costs $0.00065, so writes are unsurprisingly 5 times more expensive than reads. So even in provisioned mode, you’re still paying a 5x penalty on writes. Thus, is significant, especially for high-volume write workloads. No provisioned discount on writes for you! You’re not provisioning requests, you’re provisioning rates… Here’s the catch: provisioned capacity units are measured per second, not per million requests, like in on-demand. That tripped me up initially. Why not just provision the total number of requests? But from AWS’s perspective, it makes perfect business sense. You’re paying for the ability to handle N operations per second, whether you use that capacity or not. So if your traffic is bursty, or you’re over provisioning to avoid request throttling (more on that in a bit), you’re essentially paying for idle capacity. Put simply, you’re buying sustained capacity, even if you only need it occasionally. Just like my gym membership 😉 Reserved capacity… So here’s the deal: if you reserve capacity, you’re betting big upfront to hopefully save a bit later. If you’re confident in your baseline usage, AWS gives you the option to reserve DynamoDB capacity, just like with EC2 or RDS. It’s a prepaid 1 or 3 year commitment, where you lock in a fixed rate of reads and writes per second. And yes, it’s still a rate, not a total number of requests. One gotcha: there’s no partial upfront option; it’s pay in full or walk away. Let’s look at a simple use case to compare the pricing models… Say your workload averages 10,000 reads/sec and 10,000 writes/sec over an hour. On-Demand pricing: Writes: $22.50/hr … 10,000 * 3600 * 0.625 / 1M Reads: $4.50/hr … 10,000 * 3600 * 0.125 / 1M (5x cheaper than writes, as usual) Provisioned pricing (non-reserved): Writes: $6.50/hr … 10,000 * $0.00065 Reads: $1.30/hr … 10,000 * $0.00013 Provisioned with 1-Year Reserved: Writes: ~$2.99/hr Reads: ~$0.59/hr “Hey, where’s the reserved math?” I hear you. Let’s just say: You take the reserved pricing for 100 WCUs ($0.0128/hr) and RCUs ($0.0025/hr), divide by 730 hours in a month, divide by 12 months in a year, divide again by 100 units, multiply by your needed rate… then round it, cry a little, and paste in the “math lady” meme. Or better yet, use our calculator. My point is: Provisioned is ~3.4x cheaper than on-demand Reserved is ~7.5x cheaper than on-demand On-demand is for people who love overpaying, or loathe predicting Btw, AWS recommends on-demand for: Traffic patterns that evolve over time Spiky or batchy workloads Low utilization (drops to zero or below 30% of peak) Which is basically every real-life workload — at least for the customers of ScyllaDB. So yes, expect to pay a premium for that flexibility unless your traffic looks like a textbook sine wave and you have a crystal ball. It’s not the size of the item, but it is… Here’s another trap. It’s one that you might not hit until you use real application data…at which point you’ll immediately regret overlooking it. In DynamoDB, you don’t just pay per operation; you pay per chunk of data transferred. And the chunk sizes differ between reads and writes: Writes are billed per 1KB (Write Request Units or WRUs) Reads are billed per 4KB (Read Request Units or RRUs) So if you write a 1.1KB item, that’s 2 WRUs. Write a 3KB item? Still 3 WRUs, every 1KB (or part thereof) gets counted. Reads work the same way, just at 4KB boundaries. Read a 1KB item? 1 RRU. Read a 4.1KB item? That’s 2 RRUs. Isn’t rounding up fun? I’m sure there’s strong technical reasons for these boundaries. You can see the trap here. Combine this with the 5x cost of a write compared to a read, and things can get nasty quickly, especially if your item size straddles those thresholds without you realizing. It’s probably ok if you have a fixed item size in your schema, but definitely not ok with the types of use cases we see at ScyllaDB. For example, customers might have nested JSON or blob fields which can shrink or grow with usage. And remember, it’s actual item size, not just logical schema size. Overprovisioning, because you have to … Another pain point, and devious omission from AWS’s own calculator, is the need to overprovision when using provisioned capacity. It sounds counterintuitive, but you’re forced to overprovision – not because you want to, but because DynamoDB punishes you if you don’t. In provisioned mode, every request is subject to strict throughput limits because, if you recall earlier, a fixed rate is what you’re paying for. If you slide past the provisioned capacity, you’ll hit ProvisionedThroughputExceededException. I love the clarity of this type of exception message. I don’t love what it actually does, though: request throttling. There’s a small 300s window of burst capacity that retains unused read and write capacity. But beyond that, your app just fails. So, the best way to counter this is to overprovision. By how much? That warrants an “it depends” answer. But it does depend on your workload type. We added this functionality to our calculator so you can dynamically overprovision by a percentage, just to factor in the additional costs to your workload. Obviously, these costs can add up quickly because in practice, you’re paying for the peak even if you operate in the trough. If you don’t provision high enough capacity, your peaks risk being throttled, giving you customer-facing failures at the worst possible time. Before we move on … If there’s a recurring theme here, it’s this: DynamoDB’s pricing isn’t inherently wrong. You do pay for what you use. However, it’s wildly unforgiving for any workload that doesn’t look like a perfect, predictable sine wave. Whether it’s: The 5x write cost multiplier The 7.5x on-demand cost multiplier Opaque per-second provisioned rates Punitive rounding and artificial boundaries of item sizes Or just the need to overprovision to avoid face-planting during peak load …You’re constantly having to second guess your architecture just to stay ahead of cost blowouts. The irony? DynamoDB is branded as “serverless” and “fully managed” yet you end up managing capacity math, throttling errors, arcane pricing tiers, and endless throughput gymnastics. Having observed many of our customer’s spreadsheet forecasts (and AWS Cost Explorer exports) for DynamoDB, even mature teams running large-scale systems have no idea what the cost is…until it’s too late. That’s why we built a calculator that models real workloads, not just averages. Because the first step to fixing costs is to understand where they’re coming from. In my next blog post, I walk through some real-world examples of customers that switched from DynamoDB to ScyllaDB to show the true impact of traffic patterns, item sizes, caches and multi region topologies. Stay tuned or skip ahead and model your own workloads at calculator.scylladb.com. Model your own DynamoDB workloads on our new cost calculatorBig ScyllaDB Performance Gains on Google Cloud’s New Smaller Z3 Instances
Benchmarks of ScyllaDB on Google Cloud’s new Z3 small instances achieved higher throughput and lower latency than N2 equivalents, especially under heavy load ScyllaDB recently had the privilege of examining Google Cloud’s shiny new small shape Z3 GCE instances in an early preview. The Z3 series is optimized for workloads that require low latency and high performance access to large data sets. Likewise, ScyllaDB is engineered to deliver predictable low latency, even with workloads exceeding millions of OPS per machine. Naturally, both ScyllaDB and Google Cloud were curious to see how these innovations translated to performance gains with data-intensive use cases. So, we partnered with Google Cloud to test ScyllaDB on the new instances. TL;DR When we tested ScyllaDB on these new Z3 small shape instances vs. the previous generation of N2 instances, we found significant throughput improvements as well as reduced latencies…particularly at high load scenarios. Why the New Z3 Instances Matter Z3 is Google Cloud’s first generation of Storage Optimized VMs, specifically designed to combine the latest CPU, memory, network, and high-density local SSD advancements. It introduces 36 TB of local SSD with up to 100 Gbps network throughput in its largest shape and brings in significant software-level improvements like partitioned placement policies, enhanced maintenance configurations, and optimized Hyperdisk support. The Z3 series has been available for over a year now. Previously, Z3 was only available in large configurations (88 and 176 vCPUs). With this new addition to the Z3 family, users can now choose from a broader range of high-performance instances, including shapes with 8, 16, 22, 32, and 44 vCPUs – all built on 4th Gen Intel Xeon Scalable (Sapphire Rapids), DDR5 memory, and local SSDs configured for maximum density and throughput. The new instance types — especially those in the 8 to 44 vCPU range — allow ScyllaDB to extend Z3 performance advantages to a broader set of workloads and customer profiles. And now that ScyllaDB X Cloud just introduced support for mixed-instance clusters, it’s the perfect timing for these new instances. Our customers can use them to expand and contract capacity with high precision. Or they can start small, then seamlessly shift to larger instances as their traffic grows. Test Methodology We evaluated the new Z3 instances against our current N2-based configurations using our standard weekly regression testing suite. These tests focus on measuring latency across a range of throughput levels, including an unthrottled phase to identify maximum operations per second. For all tests, each cluster consisted of 3 ScyllaDB nodes. The Z3 clusters used z3-highmem-16-highlssd instances, while the N2 clusters used n2-highmem-16 instances with attached 6 TB high-performance SSDs to match the Z3 clusters’ storage. Both instance families come with 16 vCPUs and 128 GB RAM. The replication factor was set to 3 to reflect our typical production setup. Four workloads were tested on ScyllaDB version 2025.1.2 with vnode-based keyspaces: Read (100% cache hit) Read (100% cache miss) Write Mixed (50% reads, 50% writes) For load generation, we used cassandra-stress with 1kb row size (one column). Each workload was progressively throttled to multiple fixed throughput levels, followed by an unthrottled phase. For throttled scenarios, we aimed for sub-millisecond to ~10ms latencies. For unthrottled loads, latency was disregarded to maximize throughput measurements. Benchmark Results First off, here’s an overview of the throughput results, combined: Now for the details… 1. Read Workload (100% Cache Hit) Latency results Load N2 P99 [ms] Z3 P99 [ms] 150k 0.64 0.5 300k 1.37 0.86 450k 7.23 6.23 600k Couldn’t meet op/s 10.02 700k Couldn’t meet op/s 13.1 The Z3 cluster consistently delivered better tail latencies across all load levels. For higher loads, the N2 based cluster couldn’t keep up, so we presented only results for the Z3 cluster. Maximum throughput results Load N2 Throughput Z3 Throughput Diff % Max 569,566 1,151,739 102 Due to superb performance gains from the CPU family upgrade, the Z3 cluster achieved a staggering 102% higher throughput than the N2 did at the unthrottled level. 2. Read Workload (100% Cache Miss) Latency results Load N2 P99 [ms] Z3 P99 [ms] 80k 2.53 2.02 165k 3.99 3.11 250k Couldn’t meet op/s 4.7 Again, the Z3 cluster achieved better latency results across all tested loads and could serve higher throughput while keeping latencies low. Maximum throughput results Load N2 Throughput Z3 Throughput Diff % Max 236,528 310,880 31 With a 100% cache read workload that’s bounded by a mix of disk and CPU performance, the Z3 cluster achieved a significant 31% gain in maximum throughput. 3. Write Workload Latency results Load N2 P99 [ms] Z3 P99 [ms] 200k 3.27 3.21 300k >100 ms 4.19 Although latencies remained relatively similar under moderate load, the N2 instances couldn’t sustain them under higher loads. Maximum throughput results Load N2 Throughput Z3 Throughput Diff % Max 349,995 407,951 17 Due to heavy compactions and intensive disk utilization, the write workload also takes advantage of Z3’s advancements. Here, it achieved 17% higher throughput. 4. Mixed Workload (50% Read / 50% Write) Latency results Load N2 P99 Write [ms] Z3 P99 Write [ms] N2 P99 Read [ms] Z3 P99 Read [ms] 50k 2.07 2.04 2.08 2.11 150k 2.27 2.65 2.65 2.93 300k 4.71 3.88 5.12 4.15 450k >100 ms 15.49 >100 ms 16.13 The Z3 cluster maintained similar latency characteristics to the N2 one in lower throughput ranges. In higher ones, it kept a consistent edge since it was able to serve data reliably at a wider range. Maximum throughput results Load N2 Throughput Z3 Throughput Diff % Max 519,154 578,380 11 With a 50% read:write ratio, the Z3 instances achieved 11% higher throughput for both read and write operations. Our Verdict on the New Z3 Instances The addition of Z3 smaller shapes brings new flexibility to ScyllaDB Cloud users. Whether you’re looking to scale down while retaining high SSD performance or ramp up throughput in cost-sensitive environments, Z3 offers a compelling alternative to N2. We’re excited to support the smaller Z3 instance types in ScyllaDB Cloud. These VMs will complement the existing N2 options and enable more flexible deployment profiles for workloads that demand high storage IOPS and network bandwidth without committing to extremely large core counts. What’s Next This first round of testing found that performance improvements on Z3 become significantly more pronounced as the load scales. We believe that stems from ScyllaDB’s ability to fully utilize the underlying hardware. Moving forward, we’ll continue validating Z3 under other scenarios (e.g., higher disk utilization, large partitions, compaction pressure, heterogeneous cluster mixing) and uplift our internal tuning recommendations accordingly.Real-Time Machine Learning with ScyllaDB as a Feature Store
What ML feature stores require and how ScyllaDB fits in as fast, scalable online feature store In this blog post, we’ll explore the role of feature stores in real-time machine learning (ML) applications and why ScyllaDB is a strong choice for online feature serving. We’ll cover the basics of features, how feature stores work, their benefits, the different workload requirements, and how latency plays a critical role in ML applications. We’ll wrap up by looking at popular feature store frameworks like Feast and how to get started with ScyllaDB as your online feature store. What is a feature in machine learning? A feature is a measurable property used to train or serve a machine learning model. Features can be raw data points or engineered values derived from the raw data. For instance, in a social media app like ShareChat, features might include: Number of likes in the last 10 minutes Number of shares over the past 7 days Topic of the post Image credit: Ivan Burmistrov and Andrei Manakov (ShareChat) These data points help predict outcomes such as user engagement or content recommendation. A feature vector is simply a collection of features related to a specific prediction task. For example, this is what a feature vector could look like for a credit scoring application. zipcode person_age person_income loan_amount loan_int_rate (%) 94109 25 120000 10000 12 Selecting relevant data points and transforming them into features takes up a significant portion of the work in machine learning projects. It is also an ongoing process to refine and optimize features so the model being trained becomes more accurate over time. Feature store architectures In order to efficiently work with features, you can create a central place to manage the features that are available within your organization. A central feature store enables: A standard process to create new features Storage of features for simplified access Discovery and reuse of features across teams Serving features for both model training and inference Most architectures distinguish between two stores/databases: Offline store for model training (bulk writes/reads) Online store for inference (real-time, low-latency writes/reads) A typical feature store pipeline starts with ingesting raw data (from data lakes or streams), performing feature engineering, saving features in both stores, and then serving them through two separate pipelines: one for training and one for inference. Benefits of a centralized feature store Centralized feature stores offer several advantages: Avoid duplication: teams can reuse existing features Self-serve access: data scientists can generate and query features independently Unified pipelines: even though training and inference workloads are vastly different, they can still be queried using the same abstraction layer This results in faster iteration, more consistency, and better collaboration across ML workflows. Different workloads in feature stores Let’s break down the two very distinct workload requirements that exist within a feature store: model training and real-time inference. 1. Model training (offline store) In order to make predictions you need to train a machine learning model first. Training requires a large and high-quality dataset. You can store this dataset in an offline feature store. Here’s a run down of what characteristics matter most for model training workloads: Latency: Not a priority Volume: High (millions to billions of records) Frequency: Infrequent, scheduled jobs Purpose: Retrieve a large chunk of historical data Basically, offline stores need to efficiently store huge datasets. 2. Real-time inference (online store) Once you have a model ready, you can run real-time inference. Real-time inference takes the input provided by the user and turns it into a prediction. Here’s a look at what characteristics matter most for real-time inference: Latency: High priority Volume: Low per request but high throughput (up to millions of operations/second) Frequency: Constant, triggered by user actions (e.g. ordering food) Purpose: Serve up-to-date features for making predictions quickly For example, consider a food delivery app. The user’s recent cart contents, age, and location might be turned into features and used instantly to recommend other items to purchase. This would require real-time inference – and latency makes or breaks the user experience. Why latency matters Latency (in the context of this article) refers to the time between sending a query and receiving the response from the feature store. For real-time ML applications – especially user-facing ones– low latency is critical for success. Imagine a user at checkout being shown related food items. If this suggestion takes too long to load due to a slow online store, the opportunity is lost. The end-to-end flow from Ingesting the latest data Querying relevant features Running inference Returning a prediction must happen in milliseconds. Choosing a feature store solution Once you decide to build a feature store, you’ll quickly find that there are dozens of frameworks and providers, both open source and commercial, to choose from: Feast (open source): Provides flexible database support (e.g., Postgres, Redis, Cassandra, ScyllaDB) Hopsworks: Tightly coupled with its own ecosystem AWS SageMaker: Tied to the AWS stack (e.g., S3, DynamoDB) And lots of others Which one is best? Factors like your team’s technical expertise, latency requirements, and required integrations with your existing stack all play a role. There’s no one-size-fits-all solution. If you are worried about the scalability and performance of your online feature store, then database flexibility should be a key consideration. There are feature stores (e.g. AWS SageMaker, GCP Vertex, Hopsworks etc.) that provide their own database technology as the online store. On one hand, this might be convenient to get started because everything is handled by one provider. But this can also become a problem later on. Imagine choosing a vendor like this with a strict P99 latency requirement (e.g., <15ms P99). The requirement is successfully met during the proof of concept (POC). But later you experience latency spikes – maybe because your requirements change or there’s a surge of new users in your app or some other unpredictable reason. You want to switch to a different online store database backend to save costs. The problem is you cannot… at least not easily. You are stuck with the built-in solution. It’s unfeasible to migrate off just the online store part of your architecture because everything is locked in. If you want to avoid these situations, you can look into tools that are flexible regarding the offline and online store backend. Tools like Feast or FeatureForm allow you to bring your own database backend, both for the online and offline stores. This is a great way to avoid vendor lock-in and make future database migrations less painful in case latency spikes occur or costs rise. ScyllaDB as an online feature store ScyllaDB is a high-performance NoSQL database that’s API compatible with Apache Cassandra and DynamoDB API. It’s implemented in C++, uses a shard-per-core architecture, and includes an embedded cache system, making it ideal for low-latency, high-throughput feature store applications. Why ScyllaDB? Low latency (single-digit millisecond P99 performance) High availability and resilience High throughput at scale (petabyte-scale deployments) No vendor lock-in (runs on-prem or in any cloud) Drop-in replacement for existing Cassandra/DynamoDB setups Easy migration from other NoSQL databases (Cassandra, DynamoDB, MongoDB, etc) Integration with the feature store framework Feast ScyllaDB shines in online feature store use cases where real-time performance, availability, and latency predictability are critical. ScyllaDB + Feast integration Feast is a popular open-source feature store framework that supports both online and offline stores. One of its strengths is the ability to plug in your own database sources, including ScyllaDB. Read more about the ScyllaDB + Feast integration in the docs. Get started with a feature store tutorial Want to try using ScyllaDB as your online feature store? Check out our tutorials that walk you through the process of creating a ScyllaDB cluster and building a real-time inference application. Tutorial: Price prediction inference app with ScyllaDB Tutorial: Real-time app with Feast & ScyllaDB Feast + ScyllaDB integration GitHub: ScyllaDB as a feature store code examples Have questions or want help setting it up? Submit a post in the forum!Integrating support for AWS PrivateLink with Apache Cassandra® on the NetApp Instaclustr Managed Platform
Discover how NetApp Instaclustr leverages AWS PrivateLink for secure and seamless connectivity with Apache Cassandra®. This post explores the technical implementation, challenges faced, and the innovative solutions we developed to provide a robust, scalable platform for your data needs.
Last year, NetApp achieved a significant milestone by fully integrating AWS PrivateLink support for Apache Cassandra® into the NetApp Instaclustr Managed Platform. Read our AWS PrivateLink support for Apache Cassandra General Availability announcement here. Our Product Engineering team made remarkable progress in incorporating this feature into various NetApp Instaclustr application offerings. NetApp now offers AWS PrivateLink support as an Enterprise Feature add-on for the Instaclustr Managed Platform for Cassandra, Kafka®, OpenSearch®, Cadence®, and Valkey™.
The journey to support AWS PrivateLink for Cassandra involved considerable engineering effort and numerous development cycles to create a solution tailored to the unique interaction between the Cassandra application and its client driver. After extensive development and testing, our product engineering team successfully implemented an enterprise ready solution. Read on for detailed insights into the technical implementation of our solution.
What is AWS PrivateLink?
PrivateLink is a networking solution from AWS that provides private connectivity between Virtual Private Clouds (VPCs) without exposing any traffic to the public internet. This solution is ideal for customers who require a unidirectional network connection (often due to compliance concerns), ensuring that connections can only be initiated from the source VPC to the destination VPC. Additionally, PrivateLink simplifies network management by eliminating the need to manage overlapping CIDRs between VPCs. The one-way connection allows connections to be initiated only from the source VPC to the managed cluster hosted in our platform (target VPC)—and not the other way around.
To get an idea of what major building blocks are involved in making up an end-to-end AWS PrivateLink solution for Cassandra, take a look at the following diagram—it’s a simplified representation of the infrastructure used to support a PrivateLink cluster:
In this example, we have a 3-node Cassandra cluster at the far right with one Cassandra node per Availability Zone (or AZ). Next, we have the VPC Endpoint Service and a Network Load Balancer (NLB). The Endpoint Service is essentially the AWS PrivateLink, and by design AWS needs it to be backed by an NLB–that’s pretty much what we have to manage on our side.
On the customer side, they must create a VPC Endpoint that enables them to privately connect to the AWS PrivateLink on our end; naturally, customers will also have to use a Cassandra client(s) to connect to the cluster.
AWS PrivateLink support with Instaclustr for Apache Cassandra
To incorporate AWS PrivateLink support with Instaclustr for Apache Cassandra on our platform, we came across a few technical challenges. First and foremost, the primary challenge was relatively straightforward: Cassandra clients need to talk to each individual node in a cluster.
However, the problem is that nodes in an AWS PrivateLink cluster are only assigned private IPs; that is what the nodes would announce by default when Cassandra clients attempt to discover the topology of the cluster. Cassandra clients cannot do much with the received private IPs as they cannot be used to connect to the nodes directly in an AWS PrivateLink setup.
We devised a plan of attack to get around this problem:
- Make each individual Cassandra node listen for CQL queries on unique ports.
- Configure the NLB so it can route traffic to the appropriate node based on the relevant unique port.
- Let clients implement the AddressTranslator interface from the Cassandra driver. The custom address translator will need to translate the received private IPs to one of the VPC Endpoint Elastic Network Interface (or ENI) IPs without altering the corresponding unique ports.
To understand this approach better, consider the following example:
Suppose we have a 3-node Cassandra cluster. According to the proposed approach we will need to do the followings:
- Let the nodes listen on ports 172.16.0.1:6001 (in AZ1), 172.16.0.2: 6002 (in AZ2) and 172.16.0.3: 6003 (in AZ3)
- Configure the NLB to listen on the same set of ports
- Define and associate target groups based on the port. For instance, the listener on port 6002 will be associated with a target group containing only the node that is listening on port 6002.
- As for how the custom address translator is expected to work,
let’s assume the VPC Endpoint ENI IPs are 192.168.0.1 (in AZ1),
192.168.0.2 (in AZ2) and 192.168.0.3 (in AZ3). The address
translator should translate received addresses like so:
- 172.16.0.1:6001 --> 192.168.0.1:6001 - 172.16.0.2:6002 --> 192.168.0.2:6002 - 172.16.0.3:6003 --> 192.168.0.3:6003
The proposed approach not only solves the connectivity problem but also allows for connecting to appropriate nodes based on query plans generated by load balancing policies.
Around the same time, we came up with a slightly modified approach as well: we realized the need for address translation can be mostly mitigated if we make the Cassandra nodes return the VPC Endpoint ENI IPs in the first place.
But the excitement did not last for long! Why? Because we quickly discovered a key problem: there is a limit to the number of listeners that can be added to any given AWS NLB of just 50.
While 50 is certainly a decent limit, the way we designed our solution meant we wouldn’t be able to provision a cluster with more than 50 nodes. This was quickly deemed to be an unacceptable limitation as it is not uncommon for a cluster to have more than 50 nodes; many Cassandra clusters in our fleet have hundreds of nodes. We had to abandon the idea of address translation and started thinking about alternative solution approaches.
Introducing Shotover Proxy
We were disappointed but did not lose hope. Soon after, we devised a practical solution centred around using one of our open source products: Shotover Proxy.
Shotover Proxy is used with Cassandra clusters to support AWS PrivateLink on the Instaclustr Managed Platform. What is Shotover Proxy, you ask? Shotover is a layer 7 database proxy built to allow developers, admins, DBAs, and operators to modify in-flight database requests. By managing database requests in transit, Shotover gives NetApp Instaclustr customers AWS PrivateLink’s simple and secure network setup with the many benefits of Cassandra.
Below is an updated version of the previous diagram that introduces some Shotover nodes in the mix:
As you can see, each AZ now has a dedicated Shotover proxy node.
In the above diagram, we have a 6-node Cassandra cluster. The Cassandra cluster sitting behind the Shotover nodes is an ordinary Private Network Cluster. The role of the Shotover nodes is to manage client requests to the Cassandra nodes while masking the real Cassandra nodes behind them. To the Cassandra client, the Shotover nodes appear to be Cassandra nodes, and it is only them that make up the entire cluster! This is the secret recipe for AWS PrivateLink for Instaclustr for Apache Cassandra that enabled us to get past the challenges discussed earlier.
So how is this model made to work?
Shotover can alter certain requests from—and responses to—the client. It can examine the tokens allocated to the Cassandra nodes in its own AZ (aka rack) and claim to be the owner of all those tokens. This essentially makes them appear to be an aggregation of the nodes in its own rack.
Given the purposely crafted topology and token allocation metadata, while the client directs queries to the Shotover node, the Shotover node in turn can pass them on to the appropriate Cassandra node and then transparently send responses back. It is worth noting that the Shotover nodes themselves do not store any data.
Because we only have 1 Shotover node per AZ in this design and there may be at most about 5 AZs per region, we only need that many listeners in the NLB to make this mechanism work. As such, the 50-listener limit on the NLB was no longer a problem.
The use of Shotover to manage client driver and cluster interoperability may sound straight forward to implement, but developing it was a year-long undertaking. As described above, the initial months of development were devoted to engineering CQL queries on unique ports and the AddressTranslator interface from the Cassandra driver to gracefully manage client connections to the Cassandra cluster. While this solution did successfully provide support for AWS PrivateLink with a Cassandra cluster, we knew that the 50-listener limit on the NLB was a barrier for use and wanted to provide our customers with a solution that could be used for any Cassandra cluster, regardless of node count.
The next few months of engineering were then devoted to the Proof of Concept of an alternative solution with the goal to investigate how Shotover could manage client requests for a Cassandra cluster with any number of nodes. And so, after a solution to support a cluster with any number of nodes was successfully proved, subsequent effort was then devoted to work through stability testing the new solution, the results of that engineering being the stable solution described above.
We have also conducted performance testing to evaluate the relative performance of a PrivateLink-enabled Cassandra cluster compared to its non-PrivateLink counterpart. Multiple iterations of performance testing were executed as some adjustments to Shotover were identified from test cases and resulted in the PrivateLink-enabled Cassandra cluster throughput and latency measuring near to a standard Cassandra cluster throughput and latency.
Related content: Read more about creating an AWS PrivateLink-enabled Cassandra cluster on the Instaclustr Managed Platform
The following was our experimental setup for identifying the max throughput in terms of Operations per second of a Cassandra PrivateLink cluster in comparison to a non-Cassandra PrivateLink cluster
- Baseline node size:
i3en.xlarge
- Shotover Proxy node size on Cassandra Cluster:
CSO-PRD-c6gd.medium-54
- Cassandra version:
4.1.3
- Shotover Proxy version:
0.2.0
- Other configuration: Repair and backup disabled, Client Encryption disabled
Throughput results
Operation | Operation rate with PrivateLink and Shotover | Operation rate without PrivateLink |
Mixed-small (3 Nodes) | 16608 | 16206 |
Mixed-small (6 Nodes) | 33585 | 33598 |
Mixed-small (9 Nodes) | 51792 | 51798 |
Across different cluster sizes, we observed no significant difference in operation throughput between PrivateLink and non-PrivateLink configurations.
Latency results
Latency benchmarks were conducted at ~70% of the observed peak throughput (as above) to simulate realistic production traffic.
Operation | Ops/second | Setup | Mean Latency (ms) | Median Latency (ms) | P95 Latency (ms) | P99 Latency (ms) |
Mixed-small (3 Nodes) | 11630 | Non-PrivateLink | 9.90 | 3.2 | 53.7 | 119.4 |
PrivateLink | 9.50 | 3.6 | 48.4 | 118.8 | ||
Mixed-small (6 Nodes) | 23510 | Non-PrivateLink | 6 | 2.3 | 27.2 | 79.4 |
PrivateLink | 9.10 | 3.4 | 45.4 | 104.9 | ||
Mixed-small (9 Nodes) | 36255 | Non-PrivateLink | 5.5 | 2.4 | 21.8 | 67.6 |
PrivateLink | 11.9 | 2.7 | 77.1 | 141.2 |
Results indicate that for lower to mid-tier throughput levels, AWS PrivateLink introduced minimal to negligible overhead. However, at higher operation rates, we observed increased latency, most notably at the p99 mark—likely due to network level factors or Shotover.
The increase in latency is expected as AWS PrivateLink introduces an additional hop to route traffic securely, which can impact latencies, particularly under heavy load. For the vast majority of applications, the observed latencies remain within acceptable ranges. However, for latency-sensitive workloads, we recommend adding more nodes (for high load cases) to help mitigate the impact of the additional network hop introduced by PrivateLink.
As with any generic benchmarking results, performance may vary depending on specific data model, workload characteristics, and environment. The results presented here are based on specific experimental setup using standard configurations and should primarily be used to compare the relative performance of PrivateLink vs. Non-PrivateLink networking under similar conditions.
Why choose AWS PrivateLink with NetApp Instaclustr?
NetApp’s commitment to innovation means you benefit from cutting-edge technology combined with ease of use. With AWS PrivateLink support on our platform, customers gain:
- Enhanced security: All traffic stays private, never touching the internet.
- Simplified networking: No need to manage complex CIDR overlaps.
- Enterprise scalability: Handles sizable clusters effortlessly.
By addressing challenges, such as the NLB listener cap and private-to-VPC IP translation, we’ve created a solution that balances efficiency, security, and scalability.
Experience PrivateLink today
The integration of AWS PrivateLink with Apache Cassandra® is now generally available with production-ready SLAs for our customers. Log in to the Console to create a Cassandra cluster with support for AWS PrivateLink with just a few clicks today. Whether you’re managing sensitive workloads or demanding performance at scale, this feature delivers unmatched value.
Want to see it in action? Book a free demo today and experience the Shotover-powered magic of AWS PrivateLink firsthand.
Resources
- Getting started: Visit the documentation to learn how to create an AWS PrivateLink-enabled Apache Cassandra cluster on the Instaclustr Managed Platform.
- Connecting clients: Already created a Cassandra cluster with AWS PrivateLink? Click here to read about how to connect Cassandra clients in one VPC to an AWS PrivateLink-enabled Cassandra cluster on the Instaclustr Platform.
- General availability announcement: For more details, read our General Availability announcement on AWS PrivateLink support for Cassandra.
The post Integrating support for AWS PrivateLink with Apache Cassandra® on the NetApp Instaclustr Managed Platform appeared first on Instaclustr.
Netflix Tudum Architecture: from CQRS with Kafka to CQRS with RAW Hollow
By Eugene Yemelyanau, Jake Grice
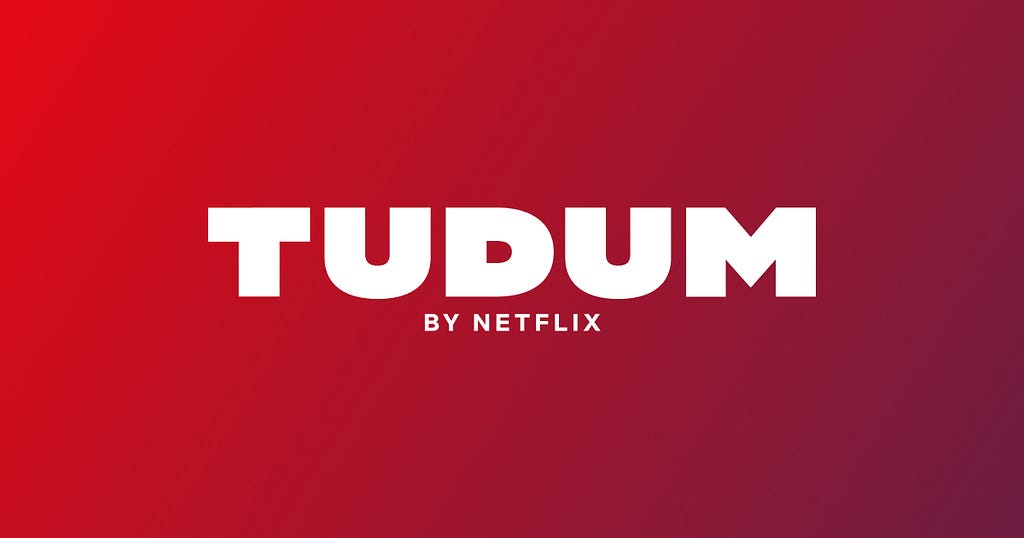
Introduction
Tudum.com is Netflix’s official fan destination, enabling fans to dive deeper into their favorite Netflix shows and movies. Tudum offers exclusive first-looks, behind-the-scenes content, talent interviews, live events, guides, and interactive experiences. “Tudum” is named after the sonic ID you hear when pressing play on a Netflix show or movie. Attracting over 20 million members each month, Tudum is designed to enrich the viewing experience by offering additional context and insights into the content available on Netflix.
Initial architecture
At the end of 2021, when we envisioned Tudum’s implementation, we considered architectural patterns that would be maintainable, extensible, and well-understood by engineers. With the goal of building a flexible, configuration-driven system, we looked to server-driven UI (SDUI) as an appealing solution. SDUI is a design approach where the server dictates the structure and content of the UI, allowing for dynamic updates and customization without requiring changes to the client application. Client applications like web, mobile, and TV devices, act as rendering engines for SDUI data. After our teams weighed and vetted all the details, the dust settled and we landed on an approach similar to Command Query Responsibility Segregation (CQRS). At Tudum, we have two main use cases that CQRS is perfectly capable of solving:
- Tudum’s editorial team brings exclusive interviews, first-look photos, behind the scenes videos, and many more forms of fan-forward content, and compiles it all into pages on the Tudum.com website. This content comes onto Tudum in the form of individually published pages, and content elements within the pages. In support of this, Tudum’s architecture includes a write path to store all of this data, including internal comments, revisions, version history, asset metadata, and scheduling settings.
- Tudum visitors consume published pages. In this case, Tudum needs to serve personalized experiences for our beloved fans, and accesses only the latest version of our content.
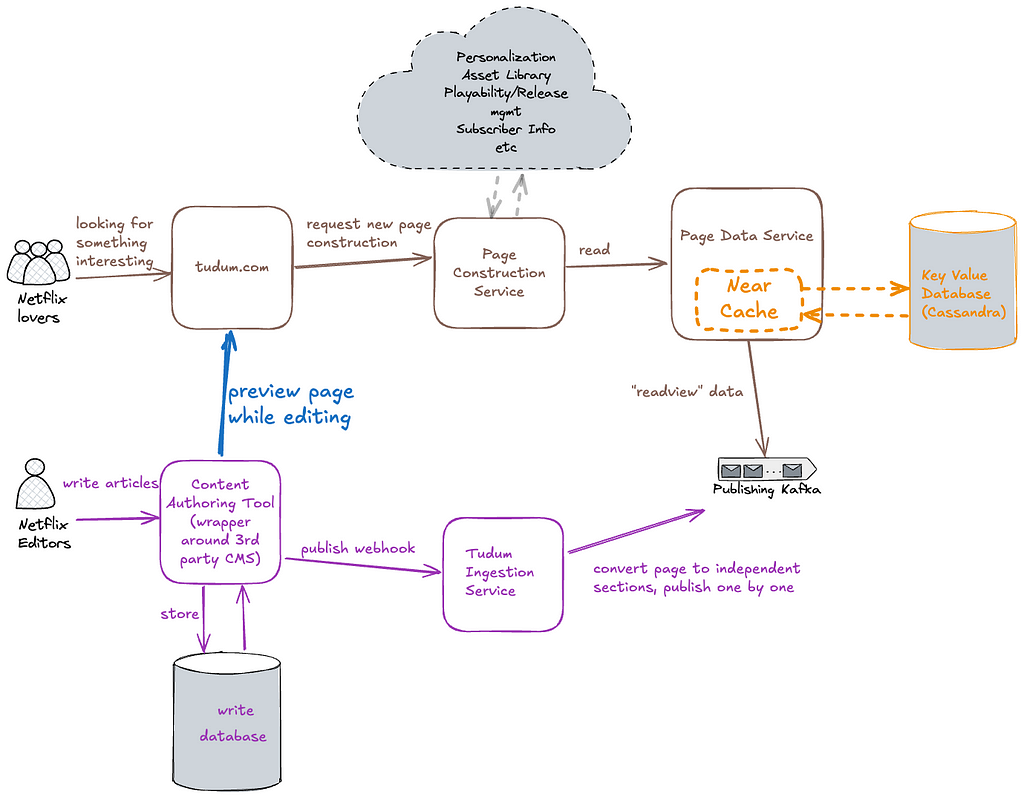
The high-level diagram above focuses on storage & distribution, illustrating how we leveraged Kafka to separate the write and read databases. The write database would store internal page content and metadata from our CMS. The read database would store read-optimized page content, for example: CDN image URLs rather than internal asset IDs, and movie titles, synopses, and actor names instead of placeholders. This content ingestion pipeline allowed us to regenerate all consumer-facing content on demand, applying new structure and data, such as global navigation or branding changes. The Tudum Ingestion Service converted internal CMS data into a read-optimized format by applying page templates, running validations, performing data transformations, and producing the individual content elements into a Kafka topic. The Data Service Consumer, received the content elements from Kafka, stored them in a high-availability database (Cassandra), and acted as an API layer for the Page Construction service and other internal Tudum services to retrieve content.
A key advantage of decoupling read and write paths is the ability to scale them independently. It is a well-known architectural approach to connect both write and read databases using an event driven architecture. As a result, content edits would eventually appear on tudum.com.
Challenges with eventual consistency
Did you notice the emphasis on “eventually?” A major downside of this architecture was the delay between making an edit and observing that edit reflected on the website. For instance, when the team publishes an update, the following steps must occur:
- Call the REST endpoint on the 3rd party CMS to save the data.
- Wait for the CMS to notify the Tudum Ingestion layer via a webhook.
- Wait for the Tudum Ingestion layer to query all necessary sections via API, validate data and assets, process the page, and produce the modified content to Kafka.
- Wait for the Data Service Consumer to consume this message from Kafka and store it in the database.
- Finally, after some cache refresh delay, this data would eventually become available to the Page Construction service. Great!
By introducing a highly-scalable eventually-consistent architecture we were missing the ability to quickly render changes after writing them — an important capability for internal previews.
In our performance profiling, we found the source of delay was our Page Data Service which acted as a facade for an underlying Key Value Data Abstraction database. Page Data Service utilized a near cache to accelerate page building and reduce read latencies from the database.
This cache was implemented to optimize the N+1 key lookups necessary for page construction by having a complete data set in memory. When engineers hear “slow reads,” the immediate answer is often “cache,” which is exactly what our team adopted. The KVDAL near cache can refresh in the background on every app node. Regardless of which system modifies the data, the cache is updated with each refresh cycle. If you have 60 keys and a refresh interval of 60 seconds, the near cache will update one key per second. This was problematic for previewing recent modifications, as these changes were only reflected with each cache refresh. As Tudum’s content grew, cache refresh times increased, further extending the delay.
RAW Hollow
As this pain point grew, a new technology was being developed that would act as our silver bullet. RAW Hollow is an innovative in-memory, co-located, compressed object database developed by Netflix, designed to handle small to medium datasets with support for strong read-after-write consistency. It addresses the challenges of achieving consistent performance with low latency and high availability in applications that deal with less frequently changing datasets. Unlike traditional SQL databases or fully in-memory solutions, RAW Hollow offers a unique approach where the entire dataset is distributed across the application cluster and resides in the memory of each application process.
This design leverages compression techniques to scale datasets up to 100 million records per entity, ensuring extremely low latencies and high availability. RAW Hollow provides eventual consistency by default, with the option for strong consistency at the individual request level, allowing users to balance between high availability and data consistency. It simplifies the development of highly available and scalable stateful applications by eliminating the complexities of cache synchronization and external dependencies. This makes RAW Hollow a robust solution for efficiently managing datasets in environments like Netflix’s streaming services, where high performance and reliability are paramount.
Revised architecture
Tudum was a perfect fit to battle-test RAW Hollow while it was pre-GA internally. Hollow’s high-density near cache significantly reduces I/O. Having our primary dataset in memory enables Tudum’s various microservices (page construction, search, personalization) to access data synchronously in O(1) time, simplifying architecture, reducing code complexity, and increasing fault tolerance.
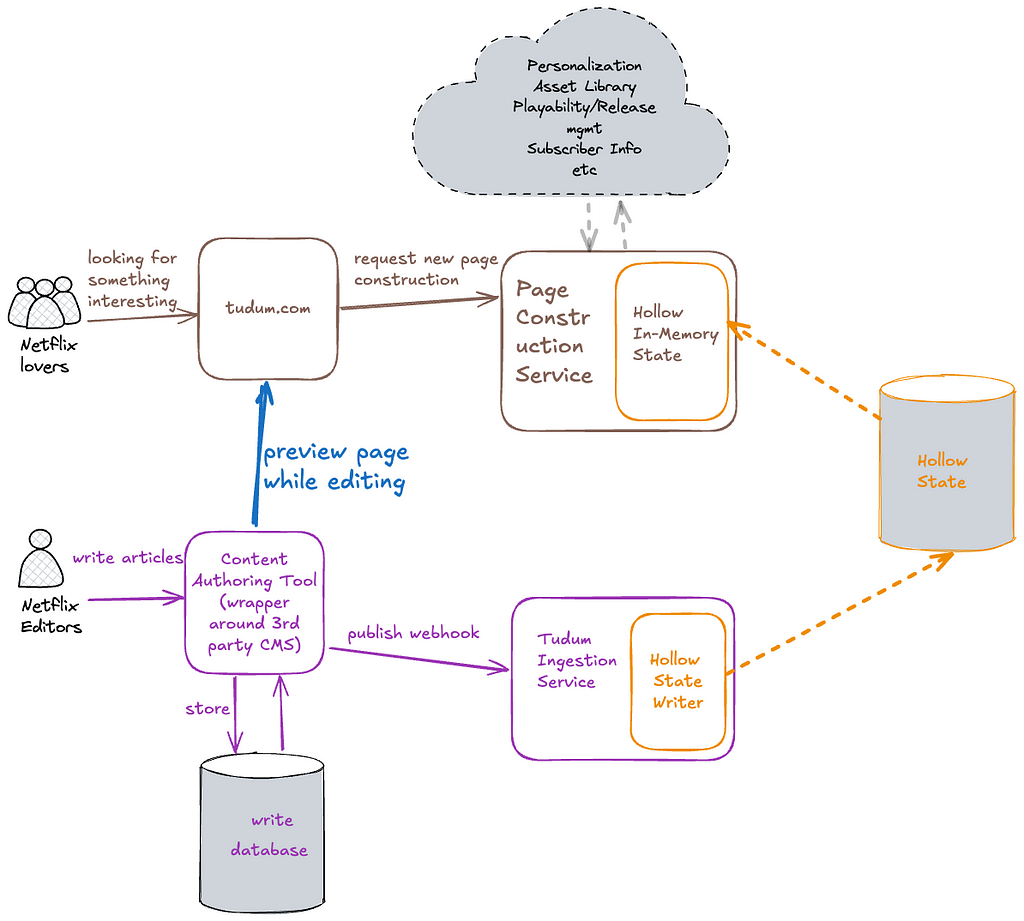
In our simplified architecture, we eliminated the Page Data Service, Key Value store, and Kafka infrastructure, in favor of RAW Hollow. By embedding the in-memory client directly into our read-path services, we avoid per-request I/O and reduce roundtrip time.
Migration results
The updated architecture yielded a monumental reduction in data propagation times, and the reduced I/O led to faster request times as an added bonus. Hollow’s compression alleviated our concerns about our data being “too big” to fit in memory. Storing three years’ of unhydrated data requires only a 130MB memory footprint — 25% of its uncompressed size in an Iceberg table!
Writers and editors can preview changes in seconds instead of minutes, while still maintaining high-availability and in-memory caching for Tudum visitors — the best of both worlds.
But what about the faster request times? The diagram below illustrates the before & after timing to fulfil a request for Tudum’s home page. All of Tudum’s read-path services leverage Hollow in-memory state, leading to a significant increase in page construction speed and personalization algorithms. Controlling for factors like TLS, authentication, request logging, and WAF filtering, homepage construction time decreased from ~1.4 seconds to ~0.4 seconds!
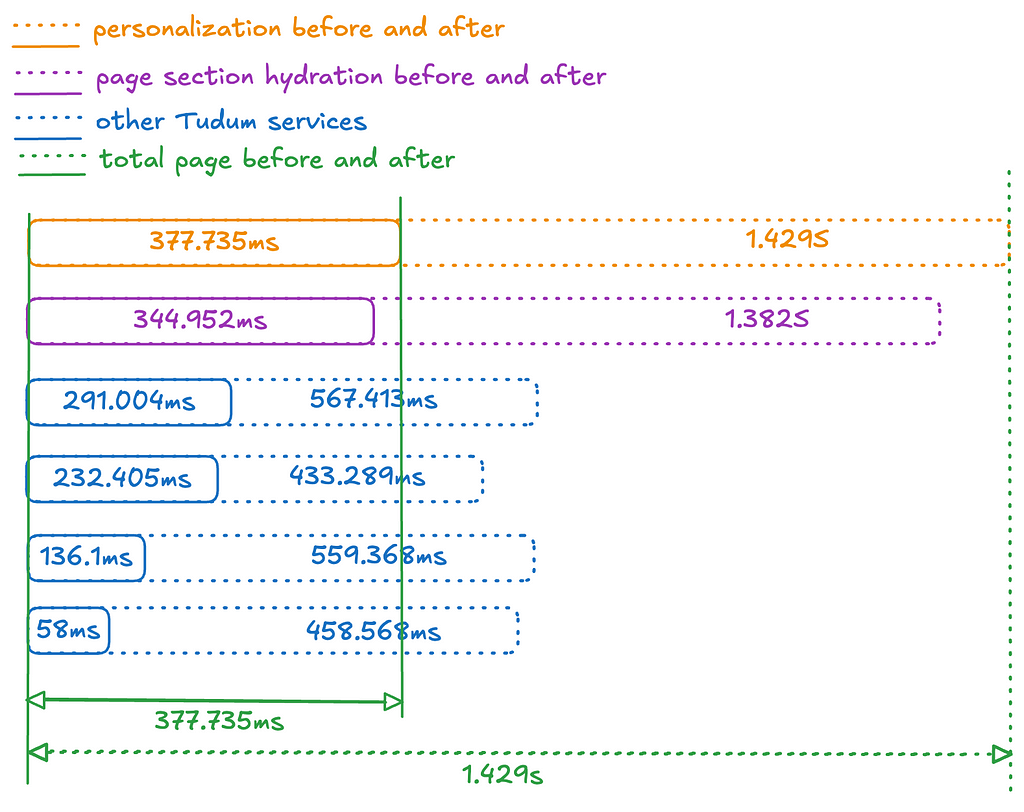
An attentive reader might notice that we have now tightly-coupled our Page Construction Service with the Hollow In-Memory State. This tight-coupling is used only in Tudum-specific applications. However, caution is needed if sharing the Hollow In-Memory Client with other engineering teams, as it could limit your ability to make schema changes or deprecations.
Key Learnings
- CQRS is a powerful design paradigm for scale, if you can tolerate some eventual consistency.
- Minimizing the number of sequential operations can significantly reduce response times. I/O is often the main enemy of performance.
- Caching is complicated. Cache invalidation is a hard problem. By holding an entire dataset in memory, you can eliminate an entire class of problems.
In the next episode, we’ll share how Tudum.com leverages Server Driven UI to rapidly build and deploy new experiences for Netflix fans. Stay tuned!
Credits
Thanks to Drew Koszewnik, Govind Venkatraman Krishnan, Nick Mooney, George Carlucci
Netflix Tudum Architecture: from CQRS with Kafka to CQRS with RAW Hollow was originally published in Netflix TechBlog on Medium, where people are continuing the conversation by highlighting and responding to this story.
Compaction Strategies, Performance, and Their Impact on Cassandra Node Density
This is the third post in my series on optimizing Apache Cassandra for maximum cost efficiency through increased node density. In the first post, I examined how streaming operations impact node density and laid out the groundwork for understanding why higher node density leads to significant cost savings. In the second post, I discussed how compaction throughput is critical to node density and introduced the optimizations we implemented in CASSANDRA-15452 to improve throughput on disaggregated storage like EBS.